Blog | AI Solutions
Next-Gen Medicine: How AI is Transforming Drug Discovery
Discover the most recent advances in drug research as artificial intelligence (AI) takes center stage in transforming the pharmaceutical industry. Learn how artificial intelligence (AI) is changing conventional research methods, hastening the creation of new drugs, and opening the door for biosimilar medications.
- Blogs
Ai in drug discovery
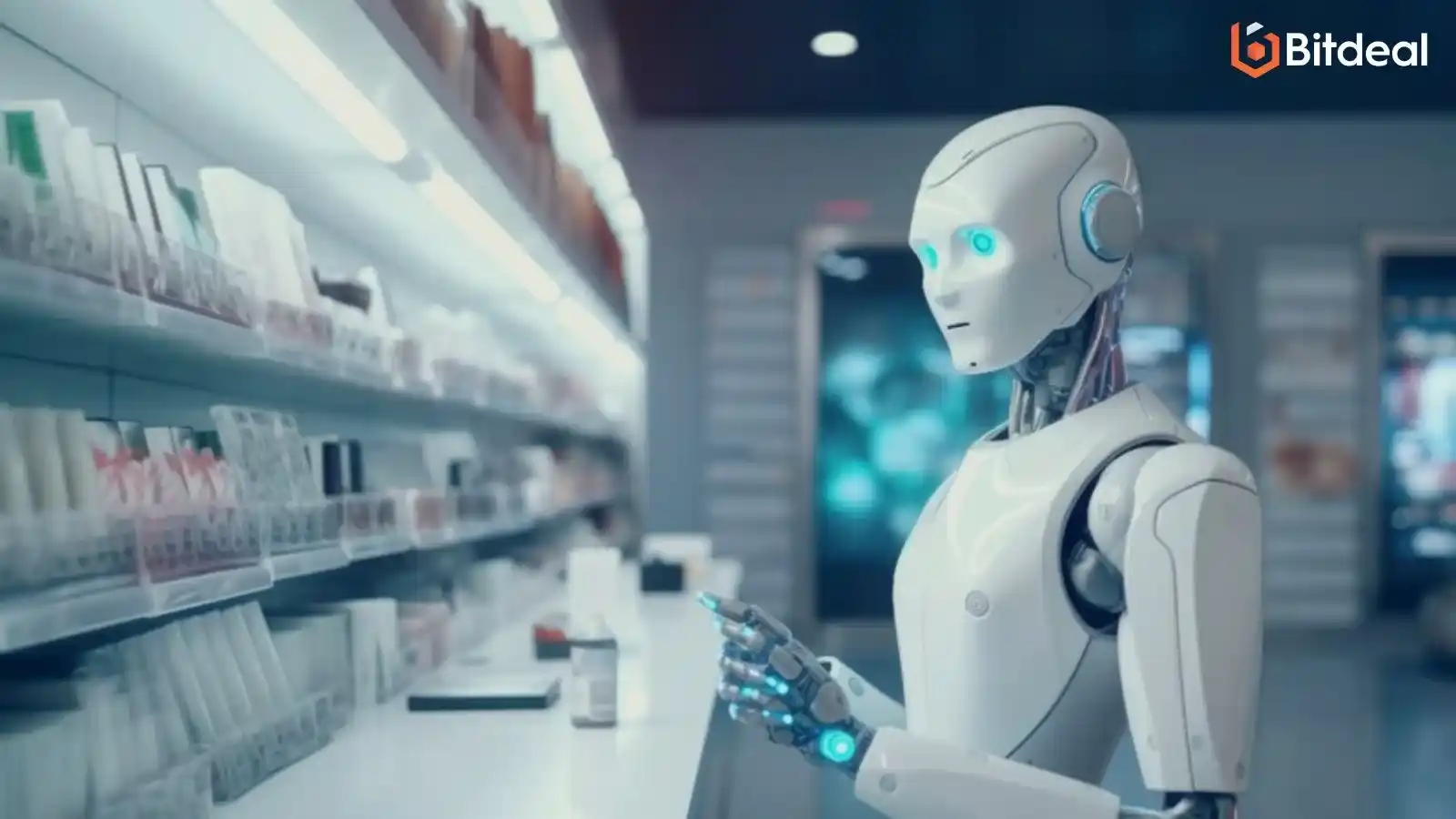
Introduction
The introduction of artificial intelligence resulted in a new era of innovation and progress in the fields of medicine and healthcare. Researchers and pharmaceutical companies may now identify possible drug candidates more quickly, streamline clinical trials, and ultimately bring life-saving medications to market more efficiently than ever before by utilizing AI's computational capacity.
The Role of AI in Predicting Drug Efficiency and Toxicity
Artificial Intelligence is a key component in modern drug discovery as it helps forecast drug toxicity and efficiency. Artificial intelligence (AI) models employ advanced algorithms and extensive datasets to evaluate complex chemical interactions and predict the efficacy of prospective medication candidates. Artificial intelligence can identify intricate patterns and linkages in genomic data, protein structures, and drug-target interactions that human researchers may miss.
AI also helps forecast the possible toxicity of drug candidates, which makes it possible to identify safety issues early and accelerate the creation of safer medications. In the end, AI's predictive powers are transforming the search for novel therapeutics through drug discovery, speeding up the procedure, and improving patient safety.
Advantages of AI in Drug Discovery
Speed: Drug development times can be greatly shortened by using AI algorithms, which can examine enormous volumes of biological, chemical, and pharmacological data at previously unheard-of speeds. It is now possible to finish tasks in a fraction of the time that they once took months or years.
Data Analysis: AI is capable of processing and analyzing a wide range of data, including chemical structures, clinical trial data, genomic data, and biological literature, to find patterns, correlations, and insights that would not be obvious to human researchers. This helps scientists choose which compounds to investigate further with greater knowledge.
Predictive Modeling: Researchers can use machine learning algorithms to develop prediction models that predict the safety and efficacy of potential drugs, allowing them to identify the most promising molecules for additional testing. Additionally, these algorithms are capable of forecasting any adverse effects or drug interactions.
Drug Repurposing: AI can determine which current medications, based on their established modes of action and possible therapeutic targets, might be repurposed for new purposes. Because repurposed medications may already have proven safety profiles, this strategy can greatly minimize the time and cost associated with generating new drugs.
Target Identification: Artificial intelligence (AI) systems are capable of analyzing protein structures and biological processes to find new therapeutic targets and comprehend disease mechanisms. This information may help create more specialized and efficient treatments.
Virtual Screening: Artificial intelligence (AI)-driven virtual screening methods may quickly sort through huge libraries of chemical compounds to find the ones that have the best chance of attaching to a target molecule, which speeds up the lead compound discovery process.
Personalized Medicine: To determine which subpopulations are more likely to respond to a specific treatment, AI algorithms can evaluate patient data, including genetic information and clinical records. This makes it possible to create patient-specific personalized medicine strategies that enhance treatment results and reduce side effects.
AI Applications in Drug Development
Target Identification and Validation: To find possible therapeutic targets linked to disease processes, AI systems examine biological data. Artificial Intelligence can detect new targets and verify their significance in the etiology of disease by examining genomic, proteomic, and metabolomic data.
Lead Identification and Optimization: Lead compound optimization and identification are made easier by AI-powered computational drug discovery systems. AI systems can anticipate the binding affinity and efficacy of drug candidates using virtual screening, molecular docking, and quantitative structure-activity relationship (QSAR) modeling, which speeds up the lead optimization process.
Drug Repurposing: AI makes it possible to find medications that are currently on the market that could be repurposed for new uses. AI systems can find novel therapeutic applications for licensed medications by evaluating sizable collections of clinical and biological data, which cuts down on the time and expense of drug development.
Clinical Trial Optimization: Artificial intelligence (AI) algorithms optimize patient recruitment and clinical trial design to increase trial efficiency and hasten the medication development process. Artificial intelligence (AI) can predict patient outcomes and identify eligible participants in clinical trials by evaluating patient data, including genetic information and electronic health records.
Predictive Modeling for Drug Safety and Efficacy: Artificial intelligence algorithms create prognostic models to evaluate the effectiveness and safety of potential medications. Artificial Intelligence (AI) can predict adverse medication reactions, identify patient subpopulations likely to respond to treatment and optimize drug dosing regimens by integrating multiple data sources, including preclinical studies, clinical trials, and real-world evidence.
Biomarker Discovery: The identification of biomarkers for prognosis, therapy response prediction, and illness diagnosis is made easier by AI-driven methods. AI algorithms find biological signals linked to treatment response and illness progression by evaluating multi-omics data and clinical outcomes. This allows for the creation of personalized medicine approaches.
Drug Formulation and Delivery Optimization: Drug formulations and delivery methods are optimized by AI algorithms to improve medication efficacy, bioavailability, and patient compliance. AI-driven drug formulation platforms provide unique drug delivery systems suited to particular therapeutic applications by evaluating pharmacokinetic and physicochemical data.
Post-Market Surveillance and Pharmacovigilance: AI systems keep an eye on real-world data to identify adverse drug reactions and guarantee drug safety after receiving regulatory approval. AI systems spot possible safety warnings and enable prompt regulatory actions to safeguard patient health by examining social media, electronic health data, and scientific publications.
Real-World Examples of AI in Drug Discovery
DeepMind's AlphaFold
DeepMind's AlphaFold AI algorithm predicts protein folding, which is critical for understanding protein function. As protein structures are usually determined after significant experimentation, AlphaFold gained notoriety in December 2020 for its correct predictions. Since it can speed up the process of finding possible drug targets and developing therapies, this discovery has important ramifications for drug discovery.
Atomwise
Atomwise is an AI-powered drug development company. Their artificial intelligence (AI) technology virtually scans millions of molecules to find promising prospects for drugs. In one noteworthy instance, Atomwise and University of Toronto researchers worked together to find two possible medications for treating Ebola virus infections. Their artificial intelligence technology made the process more faster, yielding positive outcomes in a few short weeks.
BenevolentAI
BenevolentAI uses AI to evaluate biomedical data and find novel pharmaceuticals. Using their artificial intelligence platform, BenevolentAI discovered a possible ALS cure in cooperation with University of Sheffield academics. Clinical studies for the medication candidate are presently underway, demonstrating AI's ability to find new treatments for difficult-to-treat illnesses.
Recursion Pharmaceuticals
Recursion Pharmaceuticals is using AI and machine learning to find novel therapeutics for uncommon genetic illnesses. Their approach finds possible treatment options by screening thousands of chemicals in cellular models. Recursion Pharmaceuticals used its AI-driven strategy to find a potential therapeutic candidate in one case to treat cerebral cavernous malformation (CCM), a rare vascular disorder.
Limitations of AI in Drug Discovery
Data Quality and Quantity: Large, high-quality datasets are necessary for AI algorithms to generate precise predictions. These databases, however, can be scarce in the drug discovery process, particularly for uncommon illnesses or novel targets. Furthermore, data biases like the underrepresentation of particular groups or improper experimental setups might compromise the validity and applicability of AI models.
Interpretability and Transparency: Due to the complexity and difficulty of human interpretation of their decision-making processes, many AI models utilized in drug discovery—like deep learning neural networks—are frequently referred to as "black boxes". It may be difficult for researchers to comprehend how AI-generated predictions are created as a result of this lack of interpretability, which could also raise questions regarding the efficacy and safety of discovered treatment possibilities.
Overfitting and Generalization: Overfitting is a condition in which AI models trained on certain datasets perform well on training data but struggle to generalize to new, unseen data. This might result in the detection of false-positive or non-reproducible results in drug discovery, squandering time and money on the pursuit of hazardous or ineffective medication candidates.
Future Directions for AI in the Pharmaceutical Industry
Multi-modal Data Integration: More thorough analyses and insights will be possible with the integration of many data types, including genomics, proteomics, metabolomics, electronic health records, and real-world evidence. Cutting-edge AI methods, like graph-based methods and multi-modal learning, will make it easier to integrate disparate data sources and find new treatment targets and biomarkers.
Explainable AI (XAI): Gaining confidence and acceptability in drug development will depend on resolving the interpretability issue with AI models. Future studies will concentrate on creating explainable AI methods that shed light on how AI algorithms make decisions and help scientists comprehend the biology and mechanics at play in prediction-making.
Generative Models and De Novo Drug Design: For de novo drug design, generative models like variational autoencoders (VAEs) and generative adversarial networks (GANs) show potential. These models can produce new chemical structures with the appropriate characteristics, which can lead to the identification of innovative drug candidates with enhanced specificity, safety, and efficacy.
Precision Medicine and Personalized Therapeutics: Personalized medicine plans that are based on a patient's genetic composition, illness subtype, and response to treatment can be developed thanks to AI-driven methodologies. AI algorithms will advance precision medicine efforts in drug discovery by predicting patient outcomes and identifying the best treatment plans by evaluating patient data at scale.
Drug Combination Therapies: AI will be crucial in finding synergistic medicine combinations to treat infectious illnesses and cancer, among other complex disorders. Artificial intelligence (AI) algorithms will improve combination medicines to maximize efficacy, decrease toxicity, and overcome drug resistance by examining medication-drug interactions, biological pathways, and patient data.
Biological and Chemical Space Exploration: AI-powered chemical and biological space exploration will increase the number of chemical compounds and possible therapeutic targets in the repertoire. AI systems will traverse enormous search spaces to find novel biological mechanisms and chemical scaffolds with therapeutic potential by utilizing evolutionary algorithms and reinforcement learning.
To Conclude, The combination of artificial intelligence and drug development signifies a paradigm shift in pharmaceutical research. We are on the verge of a new age in personalized medicine, where treatments are customized to each patient's needs and ground-breaking cures are more accessible than ever because of the utilization of AI-driven insights and technologies. Bitdeal, a leading AI Development Company is the perfect partner if you're looking for cutting-edge AI techniques in healthcare software development.
Key Takeaways
1. AI Transforms Drug Discovery: A new chapter of medical and healthcare innovation has begun with the advent of artificial intelligence. It facilitates more rapid identification of promising drug candidates, expedites clinical trials, and increases the efficiency with which life-saving drugs are brought to market.
2. Predicting Drug Efficiency and Toxicity: AI uses complex algorithms and large datasets to forecast the toxicity and efficacy of drugs. It can spot complex patterns in protein structures, drug interactions, and genomic data, which speeds up and secures the drug development process.
3. Advantages of AI in Drug Discovery: AI is a valuable tool for drug development because it can be used quickly, analyze large amounts of data, forecast outcomes, repurpose drugs, identify targets, do virtual screening, and provide tailored medicine—all of which can result in more effective and efficient drugs.
4. AI Applications in Drug Development: Across the whole drug development process, artificial intelligence is used in target and validation identification, lead identification and optimization, drug repurposing, clinical trial optimization, biomarker discovery, medication formulation, and post-market surveillance.
5. Real-world Examples and Limitations: Artificial intelligence (AI) is being used in drug discovery, as seen by companies like Recursion Pharmaceuticals, Atomwise, BenevolentAI, and DeepMind's AlphaFold. But to fully utilize AI's promise in pharmaceutical research, issues including interpretability, overfitting, generalization, and data quantity and quality must be resolved.
Get A Demo
We are glad to announce that, Bitdeal is making one more milestone in its journey. As Web3 technologies becomes more dominant and lucrative, bitdeal sets its footmark in AI and Gaming Space. Explore our all-new AI and Gaming Solutions below here.