Blog | AI Solutions
Understanding Retrieval Augmented Generation: An In-Depth Exploration
Delve into the intricacies of Retrieval Augmented Generation through our in-depth guide, gaining valuable insights into its complexities and real-world applications for a deeper understanding of this advanced AI methodology.
- Blogs
Detailed overview on retrieval augmented generation
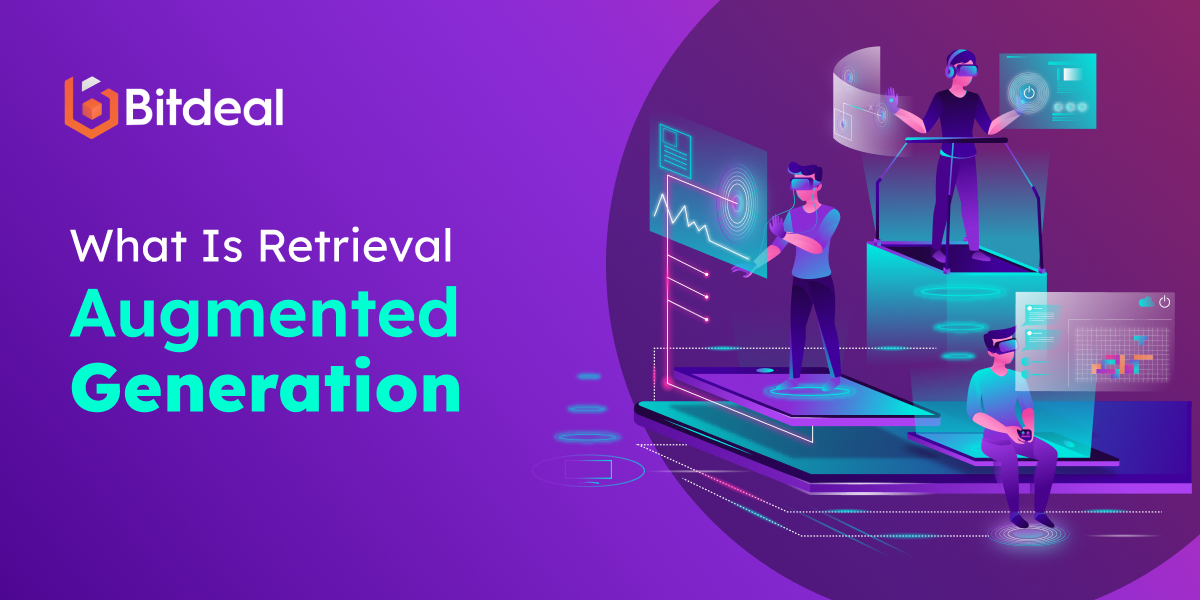
In the realm of natural language processing, Retrieval Augmented Generation (RAG) stands as a groundbreaking fusion of retrieval and generative models. This innovative approach integrates a retriever to extract contextually relevant information, which is then skillfully employed by a generative model for crafting coherent responses. This blog explores RAG's unique methodology, versatile applications, and transformative potential in advancing language understanding and generation. This blog will help you unravel the essence of Retrieval Augmented Generation and its impact on the NLP landscape.
What is Retrieval Augmented Generation (RAG)?
Retrieval Augmented Generation (RAG) is a natural language processing (NLP) model architecture that combines both retrieval-based and generative approaches. In RAG, the model uses a retriever to gather relevant information from a large dataset or knowledge base and then generates responses or outputs based on that retrieved information. This hybrid approach allows the model to benefit from the advantages of both methods.
The retriever component in RAG is responsible for efficiently searching and retrieving pertinent information from a predefined knowledge source. This retrieved information is then used by the generative model to formulate coherent and contextually relevant responses. This architecture is particularly useful in tasks where access to external knowledge is crucial for generating accurate and informative content.
RAG models have been applied to various NLP tasks, including question answering and content generation, offering a balance between the specificity of retrievers and the creativity of generative models. This combination aims to improve the overall performance and versatility of language models in understanding and generating human-like text.
How Does RAG Works?
Retrieval Augmented Generation (RAG) works by integrating two main components: a retriever and a generative model. Here's an overview of how RAG functions:
Retrieval Component:
The process begins with a retriever, which is responsible for searching and extracting relevant information from a predefined knowledge source or dataset. This knowledge source could be a large corpus of text, a database, or any other structured data.
Information Retrieval:
The retriever uses various techniques, such as keyword matching, semantic search, or embeddings, to identify and extract information that is deemed relevant to the given context or query. This retrieval step aims to gather a set of documents or passages that contain valuable information.
Generative Component:
Once the relevant information is retrieved, it is passed to the generative model. The generative model is responsible for creating coherent and contextually appropriate responses or outputs based on the retrieved information.
Response Generation:
The generative model processes the retrieved content and generates human-like responses. This can involve various natural language generation techniques, such as language modeling or transformer-based architectures, to produce text that aligns with the context and query.
Hybrid Approach:
The strength of RAG lies in its hybrid approach, combining the specificity of retrieval-based methods (retriever) with the creativity and flexibility of generative models. This allows RAG to leverage the advantages of both approaches, providing accurate and informative responses while maintaining the ability to generate novel content.
Task Applications:
RAG models can be applied to different natural language processing tasks, including question answering, content summarization, and dialogue systems. The retrieval step ensures that the model has access to relevant information, while the generative step allows it to generate responses creatively.
Reasons for Implementing Retrieval Augmented Generation (RAG):
Enhanced Context Understanding - RAG improves the model's ability to understand context by incorporating information retrieved from external sources, leading to more contextually relevant and accurate responses.
Improved Accuracy and Precision - By combining retrieval-based and generative approaches, RAG reduces ambiguity and enhances the accuracy and precision of generated responses, particularly in scenarios where context is crucial.
Versatility Across NLP Tasks - RAG is versatile and applicable to various natural language processing tasks, including question answering, summarization, and dialogue systems, providing a comprehensive solution for different use cases.
Effective Use of External Knowledge - The incorporation of a retriever component allows RAG to leverage external knowledge bases, making it beneficial for applications that require access to real-world information or industry-specific knowledge.
Customizable Retrieval Strategies - RAG offers flexibility in choosing retrieval strategies, such as keyword matching, semantic search, or embeddings, allowing developers to tailor the model to specific use cases and optimize performance.
Improved Robustness - The hybrid nature of RAG, combining retrieval and generative models, enhances model robustness by balancing the specificity of retrievers with the creativity of generative models, resulting in more reliable outputs.
Adaptability to Different Domains - The adaptability of RAG to different domains and industries makes it suitable for applications where a blend of contextual understanding and creative generation is essential, such as virtual assistants or domain-specific question answering systems.
User Experience Enhancement - Implementing RAG can enhance user experience in applications like virtual assistants or customer support by providing more accurate and contextually relevant responses, contributing to overall user satisfaction.
Benefits of Retrieval Augmented Generation:
Improved Robustness - The hybrid nature of RAG enhances model robustness by combining the specificity of retrievers with the creativity of generative models, resulting in more reliable outputs.
Contextual Relevance - Generates responses that are contextually relevant and coherent, as the model leverages information retrieved from external sources to enhance its understanding of the context.
Reduced Ambiguity - Reduces ambiguity in generated responses by incorporating external information, leading to more precise and informative answers, especially in scenarios where context is crucial.
Effective Use of Knowledge Bases - Capitalizes on knowledge bases or large datasets to enrich the model's knowledge and improve its performance in tasks requiring access to external information.
Adaptability to Various Domains - Adaptable to different domains and industries, making it suitable for applications where a blend of contextual understanding and creative generation is essential.
Enhanced User Experience - Improves user experience by providing more accurate and relevant responses in applications such as virtual assistants, customer support, and information retrieval systems.
Facilitation of Complex Tasks - Facilitates complex language tasks by leveraging the strengths of both retrieval and generative models, addressing challenges related to understanding context and generating coherent responses.
Strategic Use of External Information - Allows for strategic use of external information, providing the model with a broader knowledge base for generating content that aligns with real-world information and scenarios.
Such factors have made the importance of RAG become clear especially when noticing occasional limitations in the accuracy and currency of answers provided by LLMs. Hence, incorporating RAG into AI systems unleashes a realm of possibilities, empowering them to gather, blend, and present information in ways never seen before.
Final Thoughts:
As we explore the world of AI and RAG, Bitdeal, a top AI development company, emphasizes that it's not just about building powerful models but creating AI systems that truly understand and help people. Retrieval Augmented Generation (RAG) showcases this mission, impacting a wide range of industries and businesses.
Contact Bitdeal's expert AI team to discover the endless possibilities of RAG. Reach out to our AI consultants today to explore how RAG can benefit your business.
Get A Demo
We are glad to announce that, Bitdeal is making one more milestone in its journey. As Web3 technologies becomes more dominant and lucrative, bitdeal sets its footmark in AI and Gaming Space. Explore our all-new AI and Gaming Solutions below here.